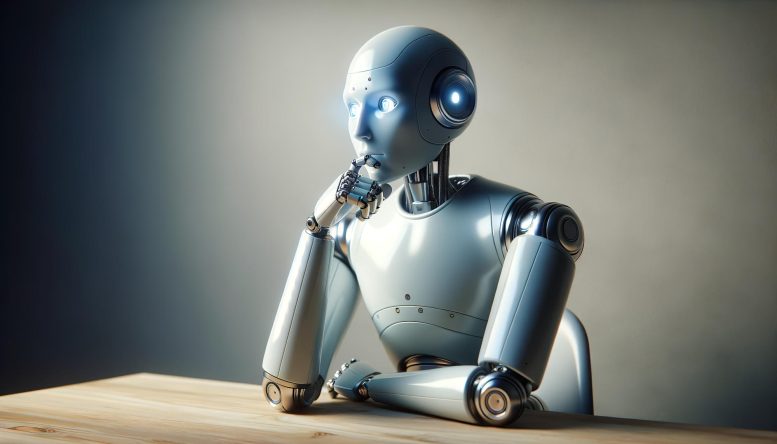
A research from the Icahn Faculty of Medication at Mount Sinai signifies that present massive language fashions will not be but efficient for medical coding, requiring additional growth and rigorous testing earlier than scientific implementation. Credit score: SciTechDaily.com
Analysis reveals its limitations in medical coding.
Researchers on the Icahn School of Medicine at Mount Sinai have discovered that state-of-the-art synthetic intelligence programs, particularly massive language fashions (LLMs), are poor at medical coding. Their research, not too long ago revealed within the NEJM AI, emphasizes the need for refinement and validation of those applied sciences earlier than contemplating scientific implementation.
The research extracted a listing of greater than 27,000 distinctive prognosis and process codes from 12 months of routine care within the Mount Sinai Well being System, whereas excluding identifiable affected person knowledge. Utilizing the outline for every code, the researchers prompted fashions from OpenAI, Google, and Meta to output essentially the most correct medical codes. The generated codes had been in contrast with the unique codes and errors had been analyzed for any patterns.
Evaluation of Mannequin Efficiency
The investigators reported that all the studied massive language fashions, together with GPT-4, GPT-3.5, Gemini-pro, and Llama-2-70b, confirmed restricted accuracy (under 50 %) in reproducing the unique medical codes, highlighting a major hole of their usefulness for medical coding. GPT-4 demonstrated the perfect efficiency, with the best precise match charges for ICD-9-CM (45.9 %), ICD-10-CM (33.9 %), and CPT codes (49.8 %).
GPT-4 additionally produced the best proportion of incorrectly generated codes that also conveyed the right which means. For instance, when given the ICD-9-CM description “nodular prostate with out urinary obstruction,” GPT-4 generated a code for “nodular prostate,” showcasing its comparatively nuanced understanding of medical terminology. Nevertheless, even contemplating these technically appropriate codes, an unacceptably massive variety of errors remained.
The subsequent best-performing mannequin, GPT-3.5, had the best tendency towards being imprecise. It had the best proportion of incorrectly generated codes that had been correct however extra normal in nature in comparison with the exact codes. On this case, when supplied with the ICD-9-CM description “unspecified adversarial impact of anesthesia,” GPT-3.5 generated a code for “different specified adversarial results, not elsewhere categorized.”
Significance of Rigorous AI Analysis
“Our findings underscore the crucial want for rigorous analysis and refinement earlier than deploying AI applied sciences in delicate operational areas like medical coding,” says research corresponding writer Ali Soroush, MD, MS, Assistant Professor of Knowledge-Pushed and Digital Medication (D3M), and Medication (Gastroenterology), at Icahn Mount Sinai. “Whereas AI holds nice potential, it should be approached with warning and ongoing growth to make sure its reliability and efficacy in well being care.”
One potential utility for these fashions within the healthcare trade, say the investigators, is automating the project of medical codes for reimbursement and analysis functions based mostly on scientific textual content.
“Earlier research point out that newer massive language fashions battle with numerical duties. Nevertheless, the extent of their accuracy in assigning medical codes from scientific textual content had not been completely investigated throughout totally different fashions,” says co-senior writer Eyal Klang, MD, Director of the D3M’s Generative AI Analysis Program. “Subsequently, our intention was to evaluate whether or not these fashions might successfully carry out the basic process of matching a medical code to its corresponding official textual content description.”
The research authors proposed that integrating LLMs with professional information might automate medical code extraction, doubtlessly enhancing billing accuracy and decreasing administrative prices in well being care.
Conclusion and Subsequent Steps
“This research sheds gentle on the present capabilities and challenges of AI in well being care, emphasizing the necessity for cautious consideration and extra refinement previous to widespread adoption,” says co-senior writer Girish Nadkarni, MD, MPH, Irene and Dr. Arthur M. Fishberg Professor of Medication at Icahn Mount Sinai, Director of The Charles Bronfman Institute of Customized Medication, and System Chief of D3M.
The researchers warning that the research’s synthetic process could not absolutely characterize real-world situations the place LLM efficiency could possibly be worse.
Subsequent, the analysis workforce plans to develop tailor-made LLM instruments for correct medical knowledge extraction and billing code project, aiming to enhance high quality and effectivity in healthcare operations.
Reference: “Massive Language Fashions Are Poor Medical Coders — Benchmarking of Medical Code Querying” by Ali Soroush, Benjamin S. Glicksberg, Eyal Zimlichman, Yiftach Barash, Robert Freeman, Alexander W. Charney, Girish N Nadkarni and Eyal Klang, 19 April 2024, NEJM AI.
DOI: 10.1056/AIdbp2300040
This analysis was supported by the AGA Analysis Basis’s 2023 AGA-Amgen Fellowship to-School Transition Award AGA2023-32-06 and an NIH UL1TR004419 award.