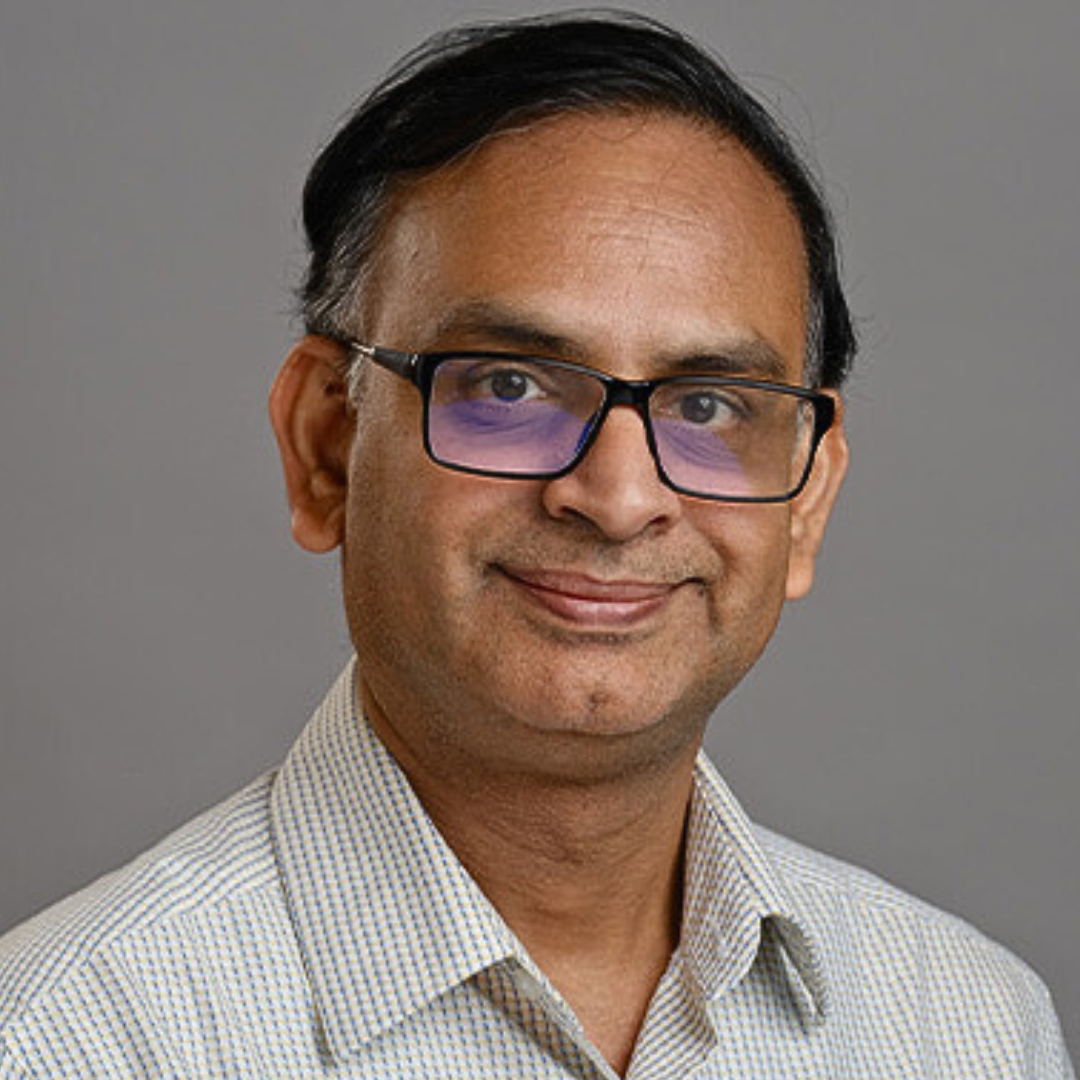
From predictive analytics to customized remedy plans, we’ve most likely all heard about AI’s potential to revolutionize medical diagnostics and remedy. As mentioned in a recent NIH study, “extra not too long ago, state-of-the-art laptop algorithms have achieved accuracies that are at par with human specialists within the area of medical sciences.”
Nonetheless, as AI algorithms more and more combine into medical gadgets and develop into extra influential in diagnoses, making certain the security of the sufferers they work together with turns into an increasing number of vital. Whereas these developments promise unprecedented precision and effectivity, there are additionally new complexities that should be navigated with nuance and foresight. We’ll take a more in-depth have a look at this significant intersection of expertise and care, exploring how healthcare professionals can navigate these complexities — upholding affected person security and privateness as a substitute of placing them in danger.
One instance to exhibit this shift comes from medical imaging. AI algorithms are being skilled to analyze mammograms for breast cancer detection past what the human eye can detect. This might probably scale back the workload for radiologists, reduce human error, and improve affected person care by enhancing early detection. Equally, AI-driven evaluation of retinal scans can identify diabetic retinopathy in its early stages, resulting in faster motion and improved affected person outcomes.
Clearly, using AI in medical imaging alone has grown exponentially, with nearly 700 FDA-approved AI algorithms across a variety of healthcare specialties as of the top of 2023 (up from simply 50 in 2013). 171 of those have been accepted between October 2022 and October 2023 alone. This speedy adoption is pushed by the promise of elevated accuracy, effectivity, and cost-effectiveness.
Medical gadgets embedded with AI capabilities can analyze huge quantities of information quickly, providing real-time diagnostic help. Monitoring gadgets that monitor very important indicators and predict potential well being points earlier than they develop into essential are understandably useful for efficient care. Due to this fact, wearable applied sciences powered by AI can allow steady well being monitoring, alerting each sufferers and healthcare suppliers to abnormalities that require consideration. This can be a vital leap in the direction of a extra proactive and customized healthcare strategy, not simply in medical system innovation however throughout all fields.
Challenges & Hurdles
Nonetheless, this speedy embrace of AI expertise additionally presents distinctive challenges. As corporations scramble to capitalize on these groundbreaking capabilities, considerations concerning information privateness, HIPAA compliance, information bias, and the interpretability of AI algorithms have surfaced.
Information privateness and HIPAA compliance are cornerstone components that ought to be constructed into any healthcare AI algorithm from the start. Whereas these algorithms depend on huge quantities of affected person information to be taught and performance successfully, this information will be anonymized and secured in accordance with HIPAA rules to prevent unauthorized access and potential breaches. Reliability relating to safety, but additionally in accuracy, uptime, and required upkeep are vital for adoption and belief as effectively, and ought to be rigorously examined by growth groups.
Bias is one other concern inside coaching datasets as a result of it could actually result in discriminatory outcomes. Coaching on sure information skewed (even unintentionally) in the direction of a particular demographic could misdiagnose people from underrepresented teams. An extension of this transparency concern, the explainability of AI algorithms is equally vital. Healthcare professionals want to know the rationale behind an AI-generated analysis to make sure belief and inform their decision-making (in different phrases, these applications can’t be opaque “black field” algorithms). This goes again to the pillar of belief, as a result of with out clear insights into how AI techniques make selections, healthcare suppliers could battle to think about and successfully make the most of these applied sciences.
Significance of Threat Evaluation & High quality Assurance Practices
Threat evaluation frameworks provide one of the efficient strategies for navigating the complexities of integrating AI with medical gadgets and diagnostics. These techniques emphasize the necessity for high-quality information at each step, verifying that the data used to coach and validate AI algorithms is correct, full, and consultant of the goal inhabitants.
Interpretability, or the flexibility to clarify the reasoning behind an AI’s output, is one other essential side. By understanding the components the algorithm thought of, healthcare professionals can assess its reliability and decide if additional investigation or human experience is required. This goes past simply healthcare professionals’ analysis of diagnoses, too — regulatory our bodies, healthcare establishments, and sufferers’ households may demand readability in how AI techniques arrive at their conclusions.
Lastly, addressing potential biases inside the information and algorithms by way of QA practices like strict information cleansing, steady testing for emergent biases, and extra are important. Threat evaluation frameworks ought to incorporate methods to determine and mitigate these biases, establishing truthful and equitable remedy for all sufferers.
Healthcare professionals should advocate for ongoing enchancment of danger evaluation practices and promote a tradition of affected person security above all else. Efficient testing (particularly in areas like biotech software program), probably incorporating automation for effectivity, ought to be an integral element of the event and deployment of AI-powered medical gadgets and diagnostics.
Proper now, the promise of this unprecedented innovation is usually met with the warranted mistrust of present techniques and suspicion of unanticipated dangers. By prioritizing early (and steady) danger evaluation and implementing thorough QA testing procedures, healthcare groups can keep on the reducing fringe of AI in diagnostics and system innovation whereas upholding affected person security and moral issues. This paves the best way for a future the place AI serves as a strong software to enhance human experience and enhance affected person outcomes throughout the healthcare panorama, with out compromising affected person care or privateness.
About Dr. Sriram Rajagopalan
Dr. Sriram Rajagopalan is the Head of Coaching & Studying Companies and Enterprise Agile Evangelist at Inflectra, the place he designs coaching curricula and gives enterprise course of consulting. He additionally serves as an Assistant Instructing Professor at Northeastern College, instructing programs on Management, Challenge Administration, Agile, and IT. Captivated with youth management, Sriram based the Projecting Leaders Of Tomorrow (PLOT) initiative and authored “Organized Widespread Sense” to help it.