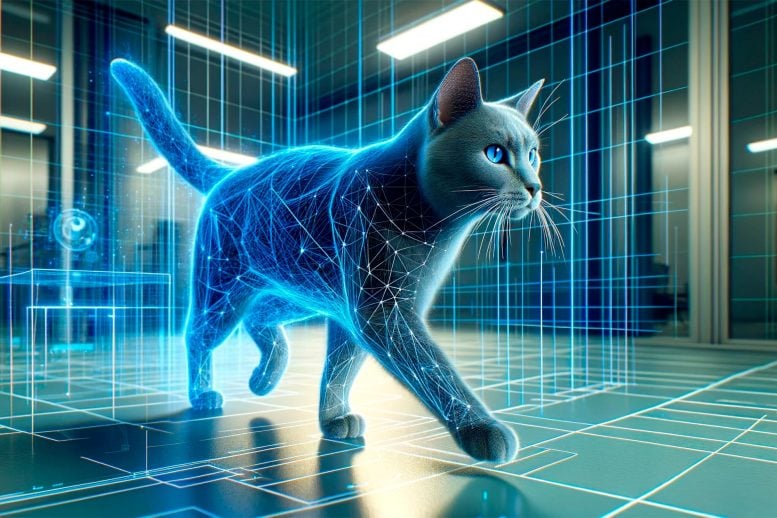
A groundbreaking AI technique created by EPFL and Harvard scientists permits for environment friendly monitoring of neurons in transferring animals, utilizing a convolutional neural community with ‘focused augmentation’. This considerably reduces handbook annotation, accelerating mind imaging analysis and deepening our understanding of neural behaviors.
EPFL and Harvard scientists develop an AI-based technique for monitoring neurons in transferring animals, enhancing mind analysis effectivity with minimal handbook annotation.
Latest advances permit imaging of neurons inside freely transferring animals. Nevertheless, to decode circuit exercise, these imaged neurons should be computationally recognized and tracked. This turns into significantly difficult when the mind itself strikes and deforms inside an organism’s versatile physique, e.g. in a worm. Till now, the scientific neighborhood has lacked the instruments to handle the issue.
Improvement of AI Methodology for Neuron Monitoring
Now, a staff of scientists from EPFL and Harvard have developed a pioneering AI technique to trace neurons inside transferring and deforming animals. The research, now printed in Nature Strategies, was led by Sahand Jamal Rahi at EPFL’s College of Primary Sciences.
The brand new technique is predicated on a convolutional neural community (CNN), which is a kind of AI that has been skilled to acknowledge and perceive patterns in photos. This includes a course of referred to as “convolution,” which appears to be like at small elements of the image – like edges, colours, or shapes – at a time after which combines all that info collectively to make sense of it and to determine objects or patterns.
The issue is that to determine and observe neurons throughout a film of an animal’s mind, many photos must be labeled by hand as a result of the animal seems very otherwise throughout time as a result of many various physique deformations. Given the range of the animal’s postures, producing a enough variety of annotations manually to coach a CNN will be daunting.
Two-dimensional projection of 3D volumetric mind exercise recordings in C. elegans. Inexperienced: genetically encoded Calcium indicator, varied colours: segmented and tracked neurons. Credit score: Mahsa Barzegar-Keshteli (EPFL)
Focused Augmentation
To handle this, the researchers developed an enhanced CNN that includes ‘focused augmentation’. The modern method mechanically synthesizes dependable annotations for reference out of solely a restricted set of handbook annotations. The result’s that the CNN successfully learns the interior deformations of the mind after which makes use of them to create annotations for brand spanking new postures, drastically decreasing the necessity for handbook annotation and double-checking.
The brand new technique is flexible, having the ability to determine neurons whether or not they’re represented in photos as particular person factors or as 3D volumes. The researchers examined it on the roundworm Caenorhabditis elegans, whose 302 neurons have made it a preferred mannequin organism in neuroscience.
Utilizing the improved CNN, the scientists measured exercise in a number of the worm’s interneurons (neurons that bridge indicators between neurons). They discovered that they exhibit advanced behaviors, for instance altering their response patterns when uncovered to totally different stimuli, equivalent to periodic bursts of odors.
Influence on Analysis
The staff has made their CNN accessible, offering a user-friendly graphical consumer interface that integrates focused augmentation, streamlining the method right into a complete pipeline, from handbook annotation to closing proofreading.
“By considerably decreasing the handbook effort required for neuron segmentation and monitoring, the brand new technique will increase evaluation throughput 3 times in comparison with full handbook annotation,” says Sahand Jamal Rahi. “The breakthrough has the potential to speed up analysis in mind imaging and deepen our understanding of neural circuits and behaviors.”
Reference: “Automated neuron monitoring inside transferring and deforming C. elegans utilizing deep studying and focused augmentation” by Core Francisco Park, Mahsa Barzegar-Keshteli, Kseniia Korchagina, Ariane Delrocq, Vladislav Susoy, Corinne L. Jones, Aravinthan D. T. Samuel and Sahand Jamal Rahi, 5 December 2023, Nature Strategies.
DOI: 10.1038/s41592-023-02096-3
Funding: École Polytechnique Fédérale de Lausanne (EPFL), Helmut Horten Stiftung, Swiss Information Science Middle