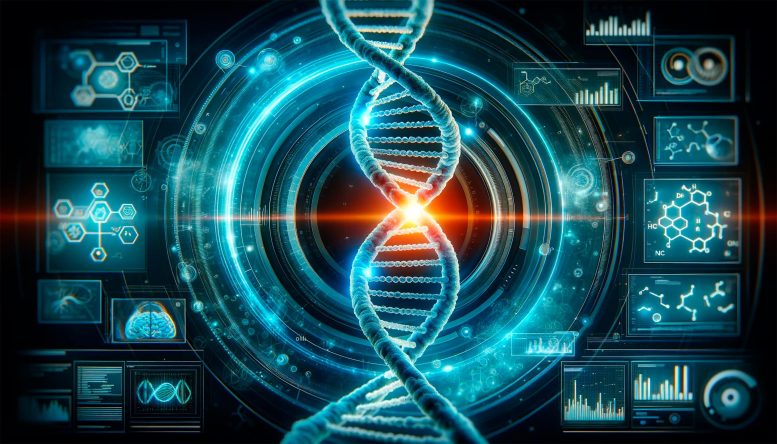
A breakthrough statistical instrument by College of Chicago researchers enhances the accuracy of discovering genetic variants linked to illnesses, providing new insights into LDL ldl cholesterol and potential remedies. Credit score: SciTechDaily.com
Statistical mannequin developed by College of Chicago researchers incorporates genome and gene expression knowledge to reliably determine causal genes.
A brand new statistical instrument developed by researchers on the College of Chicago improves the power to search out genetic variants that trigger illness. The instrument, described in a brand new paper printed January 26, 2024, in Nature Genetics, combines knowledge from genome-wide affiliation research (GWAS) and predictions of genetic expression to restrict the variety of false positives and extra precisely determine causal genes and variants for a illness.
The Challenges of GWAS
GWAS is a generally used strategy to attempt to determine genes related to a variety of human traits, together with most typical illnesses. Researchers evaluate genome sequences of a giant group of individuals with a particular illness, for instance, with one other set of sequences from wholesome people. The variations recognized within the illness group might level to genetic variants that enhance danger for that illness and warrant additional research.
Most human illnesses are usually not brought on by a single genetic variation, nonetheless. As an alternative, they’re the results of a posh interplay of a number of genes, environmental elements, and host of different variables. Because of this, GWAS usually identifies many variants throughout many areas within the genome which can be related to a illness. The limitation of GWAS, nonetheless, is that it solely identifies affiliation, not causality. In a typical genomic area, many variants are extremely correlated with one another, as a result of a phenomenon known as linkage disequilibrium. It is because DNA is handed from one era to subsequent in complete blocks, not particular person genes, so variants close by one another are typically correlated.
Advancing Past GWAS Limitations
“You will have many genetic variants in a block which can be all correlated with illness danger, however you don’t know which one is definitely the causal variant,” stated Xin He, PhD, Affiliate Professor of Human Genetics, and senior creator of the brand new research. “That’s the elemental problem of GWAS, that’s, how we go from affiliation to causality.”
To make the issue even tougher, a lot of the genetic variants are positioned in non-coding genomes, making their results troublesome to interpret. A standard technique to handle these challenges is utilizing gene expression ranges. Expression quantitative trait loci, or eQTLs, are genetic variants related to gene expression.
The rationale of utilizing eQTL knowledge is that if a variant related to a illness is an eQTL of some gene X, then X is probably the hyperlink between the variant and the illness. The issue with this reasoning, nonetheless, is that close by variants and eQTLs of different genes might be correlated with the eQTL of the gene X whereas affecting the illness instantly, resulting in a false constructive. Many strategies have been developed to appoint danger genes from GWAS utilizing eQTL knowledge, however all of them endure from this elementary drawback of confounding by close by associations. In actual fact, current strategies can generate false constructive genes greater than 50% of the time.
Innovating Genetic Analysis With cTWAS
Within the new research, Prof. He and Matthew Stephens, PhD, the Ralph W. Gerard Professor and Chair of the Departments of Statistics and Professor of Human Genetics, developed a brand new technique known as causal-Transcriptome-wide Affiliation research, or cTWAS, that makes use of superior statistical methods to cut back false constructive charges. As an alternative of specializing in only one gene at a time, the brand new cTWAS mannequin accounts for a number of genes and variants. Utilizing a Bayesian a number of regression mannequin, it will possibly weed out confounding genes and variants.
“In case you take a look at separately, you’ll have false positives, however when you take a look at all of the close by genes and variants collectively, you’re more likely to search out the causal gene,” He stated.
The paper demonstrates the utility of this new approach by learning genetics of LDL levels of cholesterol. As one instance, current eQTL strategies nominated a gene concerned in DNA restore, however the brand new cTWAS strategy pointed at a distinct variant within the goal gene of statin, a standard drug used to deal with excessive ldl cholesterol. In complete, cTWAS recognized 35 putative causal genes of LDL, greater than half of which haven’t been beforehand reported. These outcomes level to new organic pathways and potential remedy targets for LDL.
Future Instructions and Software program Availability
The cTWAS software program is now out there to download from He’s lab web site. He hopes to proceed engaged on it to increase its capabilities to include different varieties of ‘omics knowledge, reminiscent of splicing and epigenetics, in addition to utilizing eQTLs from a number of tissue varieties.
“The software program will enable individuals to do analyses that join genetic variations to phenotypes. That’s actually the important thing problem dealing with all the discipline,” He stated. “We now have a a lot better instrument to make these connections.”
Reference: “Adjusting for genetic confounders in transcriptome-wide affiliation research results in dependable detection of causal genes” 26 January 2024, Nature Genetics.
DOI: 10.1038/s41588-023-01648-9
Further authors on the research embody Siming Zhao, Wesley Crouse, Sheng Qian, and Kaixuan Luo from the College of Chicago.