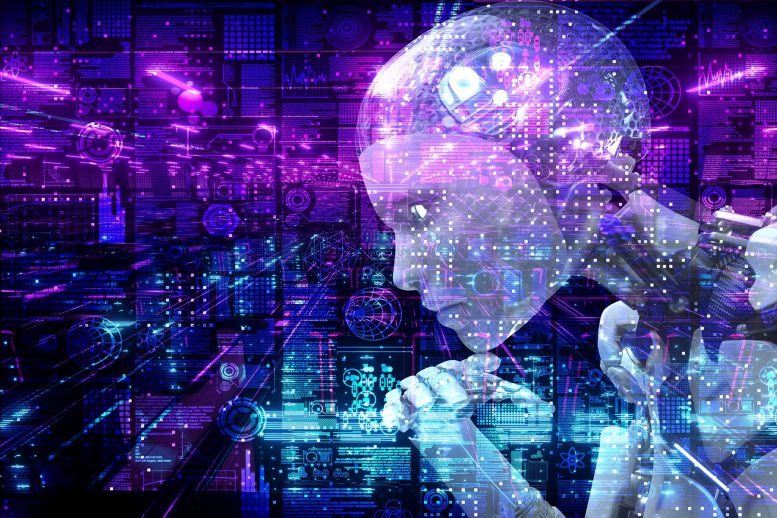
An AI mannequin developed by the Beckman Institute allows exact medical diagnoses with visible maps for rationalization, enhancing doctor-patient communication and facilitating early illness detection.
Medical diagnostics skilled, physician’s assistant, and cartographer are all truthful titles for a synthetic intelligence mannequin developed by researchers on the Beckman Institute for Superior Science and Expertise.
Their new mannequin precisely identifies tumors and illnesses in medical photos and is programmed to clarify every prognosis with a visible map. The software’s distinctive transparency permits medical doctors to simply comply with its line of reasoning, double-check for accuracy, and clarify the outcomes to sufferers.
“The concept is to assist catch most cancers and illness in its earliest levels — like an X on a map — and perceive how the choice was made. Our mannequin will assist streamline that course of and make it simpler on medical doctors and sufferers alike,” mentioned Sourya Sengupta, the examine’s lead creator and a graduate analysis assistant on the Beckman Institute.
This analysis appeared in IEEE Transactions on Medical Imaging.
Cats and canines and onions and ogres
First conceptualized within the Fifties, synthetic intelligence — the idea that computer systems can study to adapt, analyze, and problem-solve like people do — has reached family recognition, due partly to ChatGPT and its prolonged household of easy-to-use instruments.
Machine studying, or ML, is one in all many strategies researchers use to create artificially clever programs. ML is to AI what driver’s schooling is to a 15-year-old: a managed, supervised atmosphere to observe decision-making, calibrating to new environments, and rerouting after a mistake or flawed flip.
Deep studying — machine studying’s wiser and worldlier relative — can digest bigger portions of knowledge to make extra nuanced selections. Deep studying fashions derive their decisive energy from the closest pc simulations we now have to the human mind: deep neural networks.
These networks — similar to people, onions, and ogres — have layers, which makes them difficult to navigate. The extra thickly layered, or nonlinear, a community’s mental thicket, the higher it performs advanced, human-like duties.
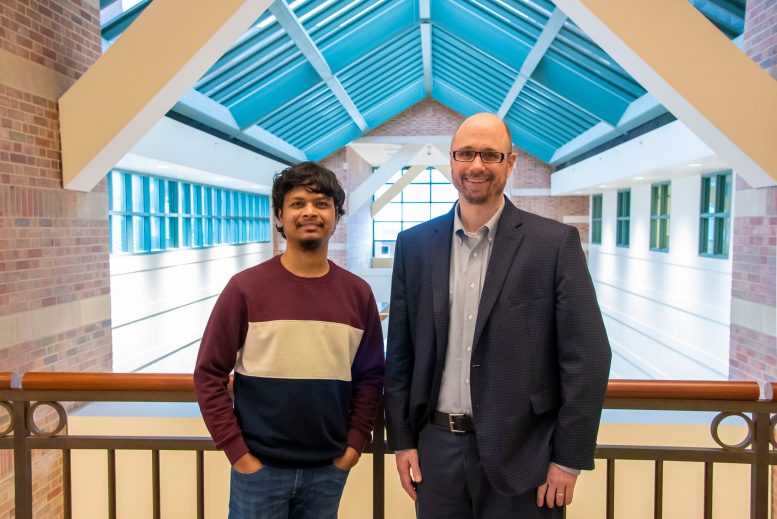
Researchers on the Beckman Institute led by Mark Anastasio (proper) and Sourya Sengupta developed a synthetic intelligence mannequin that may precisely determine tumors and illnesses in medical photos. The software attracts a map to clarify every prognosis, serving to medical doctors comply with its line of reasoning, verify for accuracy, and clarify the outcomes to sufferers. Credit score: Jenna Kurtzweil, Beckman Institute Communications Workplace
Think about a neural community educated to distinguish between photos of cats and photos of canines. The mannequin learns by reviewing photos in every class and submitting away their distinguishing options (like measurement, shade, and anatomy) for future reference. Finally, the mannequin learns to be careful for whiskers and cry Doberman on the first signal of a floppy tongue.
However deep neural networks aren’t infallible — very similar to overzealous toddlers, mentioned Sengupta, who research biomedical imaging within the College of Illinois Urbana-Champaign Division of Electrical and Pc Engineering.
“They get it proper generally, possibly even more often than not, nevertheless it may not all the time be for the proper causes,” he mentioned. “I’m certain everybody is aware of a baby who noticed a brown, four-legged canine as soon as after which thought that each brown, four-legged animal was a canine.”
Sengupta’s gripe? In case you ask a toddler how they determined, they’ll in all probability let you know.
“However you possibly can’t ask a deep neural community the way it arrived at a solution,” he mentioned.
The black field drawback
Modern, expert, and speedy as they might be, deep neural networks wrestle to grasp the seminal talent drilled into highschool calculus college students: exhibiting their work. That is known as the black field drawback of synthetic intelligence, and it has baffled scientists for years.
On the floor, coaxing a confession from the reluctant community that mistook a Pomeranian for a cat doesn’t appear unbelievably essential. However the gravity of the black field sharpens as the pictures in query turn into extra life-altering. For instance: X-ray photos from a mammogram which will point out early indicators of breast most cancers.
The method of decoding medical photos appears to be like completely different in numerous areas of the world.
“In lots of creating nations, there’s a shortage of medical doctors and an extended line of sufferers. AI will be useful in these situations,” Sengupta mentioned.
When time and expertise are in excessive demand, automated medical picture screening will be deployed as an assistive software — under no circumstances changing the talent and experience of medical doctors, Sengupta mentioned. As an alternative, an AI mannequin can pre-scan medical photos and flag these containing one thing uncommon — like a tumor or early signal of illness, known as a biomarker — for a health care provider’s evaluate. This methodology saves time and may even enhance the efficiency of the particular person tasked with studying the scan.
These fashions work nicely, however their bedside method leaves a lot to be desired when, for instance, a affected person asks why an AI system flagged a picture as containing (or not containing) a tumor.
Traditionally, researchers have answered questions like this with a slew of instruments designed to decipher the black field from the skin in. Sadly, the researchers utilizing them are sometimes confronted with an identical plight because the unlucky eavesdropper, leaning towards a locked door with an empty glass to their ear.
“It could be a lot simpler to easily open the door, stroll contained in the room, and hearken to the dialog firsthand,” Sengupta mentioned.
To additional complicate the matter, many variations of those interpretation instruments exist. Which means that any given black field could also be interpreted in “believable however completely different” methods, Sengupta mentioned.
“And now the query is: which interpretation do you imagine?” he mentioned. “There’s a probability that your alternative shall be influenced by your subjective bias, and therein lies the principle drawback with conventional strategies.”
Sengupta’s resolution? A wholly new sort of AI mannequin that interprets itself each time — that explains every resolution as an alternative of blandly reporting the binary of “tumor versus non-tumor,” Sengupta mentioned.
No water glass wanted, in different phrases, as a result of the door has disappeared.
Mapping the mannequin
A yogi studying a brand new posture should observe it repeatedly. An AI mannequin educated to inform cats from canines finding out numerous photos of each quadrupeds.
An AI mannequin functioning as a health care provider’s assistant is raised on a food regimen of 1000’s of medical photos, some with abnormalities and a few with out. When confronted with one thing never-before-seen, it runs a fast evaluation and spits out a quantity between 0 and 1. If the quantity is lower than .5, the picture just isn’t assumed to include a tumor; a numeral higher than .5 warrants a more in-depth look.
Sengupta’s new AI mannequin mimics this setup with a twist: the mannequin produces a worth plus a visible map explaining its resolution.
The map — referred to by the researchers as an equivalency map, or E-map for brief — is actually a remodeled model of the unique X-ray, mammogram, or different medical picture medium. Like a paint-by-numbers canvas, every area of the E-map is assigned a quantity. The higher the worth, the extra medically fascinating the area is for predicting the presence of an anomaly. The mannequin sums up the values to reach at its last determine, which then informs the prognosis.
“For instance, if the full sum is 1, and you’ve got three values represented on the map — .5, .3, and .2 — a health care provider can see precisely which areas on the map contributed extra to that conclusion and examine these extra absolutely,” Sengupta mentioned.
This fashion, medical doctors can double-check how nicely the deep neural community is working — like a trainer checking the work on a scholar’s math drawback — and reply to sufferers’ questions in regards to the course of.
“The result’s a extra clear, trustable system between physician and affected person,” Sengupta mentioned.
X marks the spot
The researchers educated their mannequin on three completely different illness prognosis duties together with greater than 20,000 whole photos.
First, the mannequin reviewed simulated mammograms and realized to flag early indicators of tumors. Second, it analyzed optical coherence tomography photos of the retina, the place it practiced figuring out a buildup known as Drusen that could be an early signal of macular degeneration. Third, the mannequin studied chest X-rays and realized to detect cardiomegaly, a coronary heart enlargement situation that may result in illness.
As soon as the mapmaking mannequin had been educated, the researchers in contrast its efficiency to present black-box AI programs — those and not using a self-interpretation setting. The brand new mannequin carried out comparably to its counterparts in all three classes, with accuracy charges of 77.8% for mammograms, 99.1% for retinal OCT photos, and 83% for chest X-rays in comparison with the prevailing 77.8%, 99.1%, and 83.33.%
These excessive accuracy charges are a product of the deep neural community, the non-linear layers of which mimic the nuance of human neurons.
To create such a sophisticated system, the researchers peeled the proverbial onion and drew inspiration from linear neural networks, that are less complicated and simpler to interpret.
“The query was: How can we leverage the ideas behind linear fashions to make non-linear deep neural networks additionally interpretable like this?” mentioned principal investigator Mark Anastasio, a Beckman Institute researcher and the Donald Biggar Willet Professor and Head of the Illinois Division of Bioengineering. “This work is a basic instance of how basic concepts can result in some novel options for state-of-the-art AI fashions.”
The researchers hope that future fashions will be capable to detect and diagnose anomalies all around the physique and even differentiate between them.
“I’m enthusiastic about our software’s direct profit to society, not solely by way of bettering illness diagnoses but in addition bettering belief and transparency between medical doctors and sufferers,” Anastasio mentioned.
Reference: “A Check Statistic Estimation-based Strategy for Establishing Self-interpretable CNN-based Binary Classifiers” by Sourya Sengupta and Mark A. Anastasio, 1 January 2024, IEEE Transactions on Medical Imaging.
DOI: 10.1109/TMI.2023.3348699